Artificial Intelligence is fueling a resurgence of private clouds. AI’s demand for compute resources coupled with ever-increasing public cloud costs are driving organizations to look for on-premises alternatives. Private cloud offers the flexibility and scalability of the public cloud while reining in costs and addressing data privacy concerns.
Private cloud was in vogue around the mid-2000s as organizations sought public cloud benefits along with greater control over their infrastructure and data. However, private clouds were expensive and required skill sets and management discipline that many organizations lacked.
AI is changing that equation — by some estimates, as many as 79 percent of organizations are implementing private clouds to support their AI initiatives. However, designing, implementing and maintaining a private cloud remains a complex process. Organizations may determine it makes more sense to keep AI in the public cloud or use a hybrid option that combines both public and private platforms.
The Move from Public to Private Clouds
AI may be the most significant factor driving renewed interest in private clouds, but it isn’t the only one. In Barcley’s CIO Survey 2024, a whopping 83 percent of CIOs said they plan to move workloads from the public cloud to a private cloud. That’s a big change from the first half of 2020, when just 23 percent of CIOs were planning to repatriate workloads.
Repatriation has been a growing trend in recent years. The need to move swiftly during the pandemic often forced companies to “lift and shift” workloads to the cloud before they had the opportunity to fully evaluate their options. That often leads to cloud bill shock — according to a recent report from Wasabi, 72 percent of new cloud spend in 2022 and 2023 was over budget, typically due to unexpected fees.
Cost isn’t the only reason for repatriating cloud workloads. In some cases, network bottlenecks create unexpected problems for latency-sensitive workloads. Sometimes, providers simply fail to meet expected service levels. Some workloads have additional security and compliance demands that are better handled in-house.
Using Private Clouds for AI
Security and compliance concerns are key drivers for bringing AI workloads back on-premises. As more organizations put AI applications into production, they recognize the need to ensure data privacy and security. Private clouds provide greater control over data used to train and operate AI applications.
Private clouds can improve AI performance through GPU virtualization, which allows multiple virtual machines to share a single physical graphics processing unit (GPU). This enables efficient use of GPU resources and hardware. By moving AI into a private cloud, organizations also eliminate the latency that comes with transferring data to and from the public cloud.
Additionally, the private cloud model can optimize costs, particularly for AI workloads with consistently high resource usage. Although the private cloud comes with higher upfront costs, it provides for more predictable costs over time. It also allows organizations to use specialized hardware that’s not readily available in the public cloud.
Private Cloud Challenges
Building a private cloud for AI is not easy, requiring skills and expertise across a wide range of disciplines. Organizations must integrate diverse infrastructure components and optimize GPU utilization to maximize performance and cost efficiency. The private cloud must be highly reliable and available to minimize the operational and financial impact of downtime.
The cost and complexity of the private must be weighed against its data sovereignty and predictability benefits. Some organizations may determine that the public cloud is the better alternative, even with potential privacy and security concerns. Many will also adopt a hybrid model, maintaining some workloads in the public cloud while moving others on-premises.
Technologent’s hybrid IT, cloud and AI teams are here to help you evaluate the options and determine the right platform for your AI workloads. If you opt for the private cloud, we can help you build out the infrastructure, monitoring and logging tools, optimization engines and other components you’ll need. Let us help you select and implement the best model to create a secure, high-performance, and cost-efficient foundation for AI.
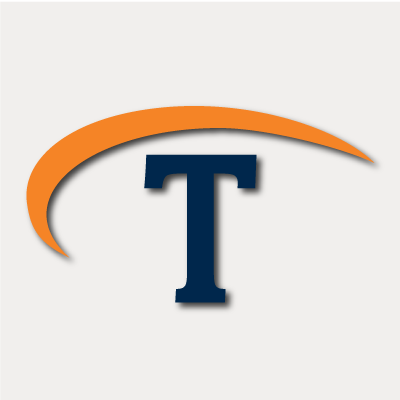
May 2, 2025
Comments