People are constantly connected to the corporate network, often running multiple applications simultaneously. Data is constantly flowing to and from a seemingly infinite number of sources. Users expect to be able to analyze this data in real time and run applications without the slightest performance disruption.
The complexity of IT combined with increasing performance demands has led to the creation of algorithmic IT operations (AIOps) platforms. According to Moogsoft, a leading AIOps vendor, AIOps, a term recently coined by Gartner, refers to solutions that use artificial intelligence and machine learning to automate tasks and processes that have traditionally required human intervention.
Although AIOps evolved from IT operations analytics (ITOA), the two terms are different. ITOA involves monitoring systems and collecting and analyzing primarily operational data from multiple sources. While ITOA focuses on historical data, AIOps allows you to visualize and analyze network conditions in real time, pinpoint problems in seconds, and automatically address problems in minutes. Moogsoft also states that using a variety of algorithms, AIOps is capable of analyzing data, performance and activity from disparate sources and provide continuous insight into both business processes and IT operations.
AIOps use cases related to IT monitoring include automated behavior prediction. By monitoring the behavior of applications, infrastructure and users, organizations can predict events that can affect availability and performance. AIOps can also be used to conduct causal analysis, applying analytics to a broad set of data to identify and compare probable root causes of issues.
On the business operations side, new opportunities and revenue streams can be identified by analyzing both IT and business data to determine what types of behavior yield the best business outcomes. AIOps can also provide data-driven recommendations based upon both real-time and historical data to inform decision-making.
Enterprises have taken notice of these capabilities and use cases, and adoption of AIOps is on the rise. Gartner estimates that about half of all global enterprises will be actively using AIOps by 2020, compared to less than 10 percent today.
There are five key components of an AIOps platform. The first is the monitoring ecosystem This provides visibility and creates telemetry across the physical and virtual stack, which is critical to maintaining high performance. However, it can also create a lot of “noise.” This noise is reduced by the expert system, which also provides real-time service insights so issues can be proactively addressed. The system of record manages service requests and trouble tickets and stores knowledge for future reference. The system of automation runs resolution scripts and streamlines repetitive tasks from common incidents. The data lake maintains all data for forensic diagnostics and other forms of deeper analysis.
AIOps uses artificial intelligence to enrich and group events, and to archive incidents based upon the nature of the disruption. This data is used to determine the best possible solution. Organic machine learning capabilities feed all identified symptoms and diagnoses into the artificial intelligence engine to enrich future uses and reduce operational expenses. A time series database uses analytics to extract meaningful data characteristics and statistics. Time series analysis also identifies outliers used to automatically create events, while time series forecasting predicts future values.
As IT complexity and performance demands continue to increase, organizations are starting to use AIOps solutions to manage and optimize both IT and business operations. Just as importantly, IT teams can leverage AIOps to identify the cause of incidents that impact the delivery of services, and then address these issues before they cause costly disruptions.
Tags:
IT Automation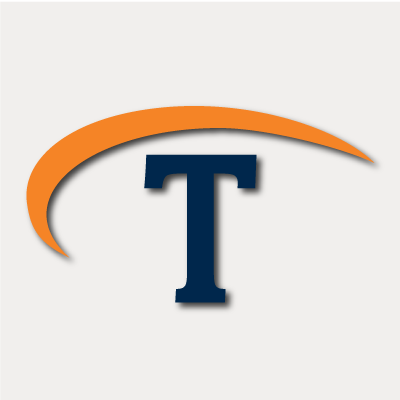
November 4, 2016
Comments