Organizations today collect and analyze staggering amounts of data in efforts to make better decisions, identify opportunities, improve processes, optimize costs and reach new customers. Turning raw data into meaningful insights is notoriously challenging, however.
The issue is that data is not homogenous — it comes in various formats and structures tailored to specific use cases and purposes. Plus, it comes from varying sources, ranging from relational databases and cloud storage to financial and enterprise resource planning systems.
In many cases, much of that data is functionally useless. Collections of structured, unstructured and object data are stored in isolated silos scattered across various systems, departments and platforms. On average, enterprise organizations are only able to leverage about a third of the data available to them.
This is driving increased uptake of data integration solutions that convert data into a unified and coherent format. In a new survey by the Omdia research firm, 71 percent of IT leaders report they plan to invest in data integration in 2023.
While data integration techniques help eliminate inconsistencies and create a single source of truth, it is a complex undertaking. Poorly developed processes can result in errors that lead to data loss or inaccuracies.
Following are four key factors that can impact integration efforts:
Data Growth
The sheer amount of data being collected makes integration an immense challenge. Aggregating and normalizing massive datasets can be a slow and resource-intensive process requiring human involvement. It can take up to three months to complete a large integration project — far too long to meet the real-time data requirements of most companies today. In a recent Wakefield Research survey of data professionals, 80 percent said data is growing faster than their ability to derive value from it.
Data Sources
According to IDG Research, the average company collects data from more than 400 sources. More than 90 percent of those surveyed said working with incompatible data formats makes it difficult to complete data analytics and digital transformation initiatives. A third said they have trouble simply connecting to multiple data sources.
Data Quality
Nonstandard and poor-quality data leads to faulty analyses and misguided decisions. According to Wakefield Research, 74 percent of data pros said they experience data quality issues with projects “all or most of the time.” In a separate study, more than half of companies said data quality issues stalled artificial intelligence projects. In many cases, quality issues arise from manual integration processes. Manually converting data with varying formats, units of measure and naming conventions increases the likelihood of errors that degrade data quality.
Streaming Data
Internet of Things (IoT) sensors, video streaming services, logistics applications and network telemetry services are among the many sources of continuous, high-speed data streams. The speed and volume of these streams make it extremely challenging to capture, store and process data quickly enough to prevent bottlenecks. To keep up, organizations must integrate data as it flows in real time. That requires a shift from batch processing, in which data is processed in predefined chunks, to continuous data pipelines. Continuous data pipelines use automation to ensure data is quickly and consistently ingested, processed, transformed and loaded to intended targets.
Conclusion
To accommodate the real-time nature of today’s business environment, organizations need to be able to create a single, unified view of data of differing structures from diverse sources. That is not a simple undertaking, however, particularly for organizations with limited IT resources. The data management pros at Technologent can help. Contact us to discuss ways to glean insights from your data resources.
Tags:
data management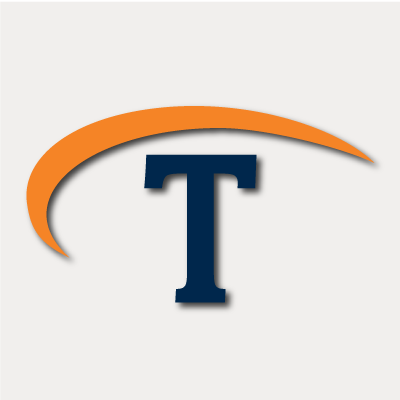
September 8, 2023
Comments