In the typical IT environment, data is trapped in multiple silos that are difficult to access and use. Because it originates from multiple sources, it is inconsistent and poorly managed. These challenges are amplified with AI and analytics.
Organizations in every industry are seeking to capitalize on the goldmine of data they create, capture and store. However, AI and analytics tools rely upon high-speed access to data to deliver the insights that enable better decision-making. Traditional data management tools used to connect disparate data stores cannot deliver the performance needed to meet these demands.
Data orchestration can help integrate data across multiple silos and locations. This technology adds an orchestration layer to storage platforms, eliminating the need to manage the flow of data manually. Unstructured data is moved automatically to a centralized repository where it is cleaned, integrated and enriched. Organizations gain the “single source of truth” they need for effective AI and analytics.
Drawbacks of Traditional ETL Tools
Extract, transform and load (ETL) tools have traditionally been used to consolidate data from disparate applications and storage platforms. This process begins with reading data from a resource and downloading the desired subset. The extracted data must be cleaned and transformed into a standard format. The extracted data must then be delivered to the target application or database.
All of this must be carefully planned and executed in the proper order, keeping in mind that problems may occur at any phase. In light of that, data is often moved to a staging area after each phase so that it can be recovered without restarting the entire process. Once these steps are completed, the data must be tested to ensure that it contains the expected values and conforms to the proper patterns. Any data that fails the validation test must be identified for analysis and correction.
Benefits of Data Orchestration
Data orchestration performs all these steps automatically according to predefined policies, dramatically increasing the speed and accuracy of data consolidation. It eliminates the need for the individuals responsible for querying data stores to process those requests, enabling more timely data analysis. Data is placed when and where it’s needed across decentralized and hybrid storage environments.
Data orchestration also increases the value of data by improving data governance. It provides a more holistic view of data across its lifecycle, giving organizations more insight into how data is managed. This enables them to improve data quality, prevent inaccurate data from being used for analysis, and optimize their storage, archival and backup practices.
It also boosts security, privacy and regulatory compliance. When organizations have a better handle on what data is stored where, they can take steps to protect sensitive information. They can also meet strict guidance for data security and respond quickly to requests from users who want to opt-out or delete their personal information.
Data Orchestration Challenges
Integrating data orchestration tools with every existing data store can be challenging. The orchestration tool must be manually connected with each repository, and the integrations must be tracked as the IT environment changes.
Data orchestration can also expose data quality issues that are difficult to overcome. Data that exists in silos is subject to the quality practices of the team responsible for capturing and managing it. Inaccuracies are commonplace. Additionally, there will likely be inconsistencies and duplication across the various silos. Organizations must ensure that data is accurate and properly cleaned before using it for AI and analytics.
Still, the benefits of data orchestration outweigh the challenges. Ensuring that data is properly captured and preserved and orchestrating its flow to AI and analytics tools can lead to faster decision-making and new insights that drive the business forward.
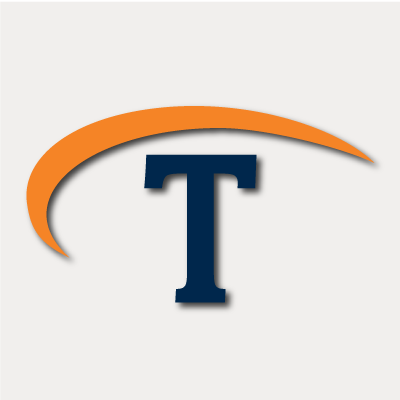
August 16, 2024
Comments